Doctors could soon evaluate Parkinson’s disease by having patients do one simple thing—sleep. A new study led by MIT researchers trains a neural network to…
Doctors could soon evaluate Parkinson’s disease by having patients do one simple thing—sleep. A new study led by MIT researchers trains a neural network to analyze a person’s breathing patterns while sleeping and determine whether the subject has Parkinson’s. Recently published in Nature Medicine, the work could lead to earlier detection and treatment.
“Our goal was to create a method for detecting and assessing Parkinson’s disease in a reliable and convenient way. Inspired by the connections between Parkinson’s and breathing signals, which are high-dimensional and complex, a natural choice was to use the power of machine learning to diagnose and track the progression,” said lead author Yuzhe Yang, a PhD student at MIT’s Computer Science & Artificial Intelligence Laboratory.
While notoriously difficult to pinpoint, Parkinson’s has become the fastest-growing neurological disease globally. About one million people in the US and 10 million worldwide are living with it. Despite these numbers, there isn’t one specific test for a speedy or definitive diagnosis.
As a progressive disorder, Parkinson’s often begins with subtle symptoms such as a slight hand tremor. It affects the nervous system and eventually leads to uncontrollable movements, shaking, stiffness while walking, and balance issues. Over time speech can become slurred and facial expressions fade away.
Neurologists often review a patient’s symptoms and medical history and rely on ruling out other illnesses based on imaging and lab work before diagnosing Parkinson’s. But symptoms vary and mimic several other disorders, which can lead to misdiagnosis and a delay in medical treatment. Early detection could help patients receive medications that are more effective when administered during the onset of Parkinson’s.
According to the authors, a correlation between nocturnal breathing and Parkinson’s was noted in 1817 by James Parkinson. A British medical doctor, he was the first to describe six individuals with symptoms of the disease he called paralysis agitans, which was later renamed.
Other research also found that brain stem degeneration in areas controlling patient breath occurs years earlier than motor skills symptoms and could be an early indicator of the disease.
The researchers saw an opportunity to employ AI, a powerful tool for detecting patterns and helping with disease diagnosis. They trained a neural network to analyze breathing patterns and learn those indicative of Parkinson’s.
The study dataset sampled 757 Parkinson’s patients and 6,914 control subjects, totaling 120,000 hours of sleep over 11,964 nights. The team trained the neural network model on several NVIDIA TITAN Xp GPUs using the cuDDN-accelerated PyTorch deep learning framework.
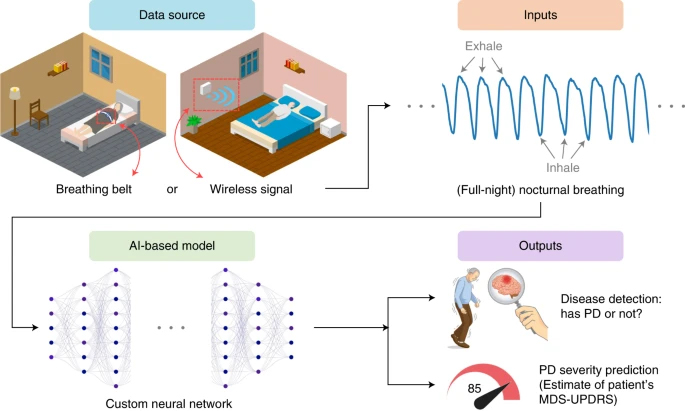
Figure 1. Overview of the AI model for Parkinson’s disease diagnosis and disease severity prediction from nocturnal breathing signals
A large amount of data came from a wireless radio transmitter the researchers developed. Similar in appearance to a Wi-Fi router the device emits radio waves and captures changes in the environment, which includes the rise and fall of a person’s chest. A neural network analyzes the patterns and determines whether Parkinson’s is present in the sample.
The AI model, deployed using NVIDIA TITAN Xp GPUs, is nearly 80% accurate in detecting Parkinson’s cases and 82% accurate in making a negative diagnosis. The algorithms can also determine the severity of Parkinson’s and track disease progression over time.
The work has the potential to speed up drug development with the newly found digital biomarkers for both diagnostics and tracking progression. Using AI models capable of detecting subtle patient changes and responses to new therapeutics could accelerate clinical trials, reduce costs, and inform more effective treatments.
It could also offer more accessible and equitable health care options to people beyond urban centers where specialists often practice medicine.
According to Yang, the team hopes to make the model more robust and accurate by collecting and testing data on more diverse populations and patients globally. They also envision use cases for the model to detect diseases beyond Parkinson’s.
“We believe there are chances to apply the method to detect other neurological diseases, for example, Alzheimer’s disease. The key problem is we need to collect a large and diverse dataset to carry out model training and evaluation for rigorous validation,” said Yang.
Contact [email protected] for information about access to the code for noncommercial purposes.
Read the research Artificial intelligence-enabled detection and assessment of Parkinson’s disease using nocturnal breathing signals.