Edge computing is a new paradigm shift for organizations, this article looks at the cost factors for rolling out these solutions.
The growth in Internet of Things (IoT) and enterprise adoption of AI has led to a renewed focus on edge computing. Organizations are looking to capitalize on the data these IoT devices produce at the edge and solve challenges that were previously impossible with centralized data centers or cloud computing.
When combined edge computing and AI, also known as edge AI, is used for real-time inference that powers digital transformation of business processes. Edge AI is a core technology for intelligent spaces that drive efficiency, automate workflows, reduce cost, and improve overall customer experience.
Today, organizations in all industries are in process of rolling out edge AI solutions—on factory floors, in retail stores, on oil rigs, and within autonomous machines.
As with any new IT initiative, getting the full benefits of edge computing requires careful planning to build a platform that will meet the needs of today and any expansion in the future.
What is edge computing?
In a broad sense, edge computing is used to refer to anything outside of the data center or cloud. More specifically, it is the practice of moving compute power physically closer to where data is generated, usually an IoT device or sensor.
Compared to cloud computing, edge computing delivers lower latency, reduced bandwidth requirements, and improved data privacy. There are different types of edge computing, often broken down based on their use case, networking requirements, or location. Content delivery networks, factory inspections, frictionless stores, and robotics are all considered examples of edge computing.
Despite the differences, the benefits remain the same: low latency, reduced bandwidth requirements, and data privacy.
How much can edge computing cost?
The cost of edge computing varies wildly depending on scale, data, location, and expertise. Overall costs can increase or decrease depending on the infrastructure currently in place at edge environments. Figure 1 shows a few key factors.

Infrastructure costs
One of the first considerations for deploying AI applications to the edge are the systems and sensors needed to support the use case. Generally, there is already some sort of infrastructure in place that must be accounted for before adding new hardware or software. The most common components of edge infrastructure are the sensors, compute systems, and network.
Sensors
Both the number and type of sensors affect the cost to an organization. Many organizations have already made investments in IoT sensors, reducing the overall investment required for an edge computing roll-out. When adding sensors, single-use scanners can add up quickly.
Cameras are some of the most versatile edge devices that give you the ability to run multiple applications simultaneously. Other sensors include microphones, barcode scanners, or RFID-enabled smart tags.
Compute systems
Compute at the edge can run on a simple embedded device that costs hundreds of dollars up. Or it can run to a half or even full rack of servers for hundreds of thousands of dollars. Compute systems are sized based on the amount of data that is being collected and processed, the complexity of one or more AI models, and the number of inferences being run at any given time.
When building out compute at the edge, it is important to consider both existing and future edge use cases that will run in the environment. Some applications can run on CPU-only systems, while others require or can greatly benefit from GPUs.
Embedded devices or even single GPU systems are cheaper up front. However, if your plan is to run multiple AI applications, a single system with multiple GPUs that can run more than one workload provides cost savings. It will also generally be more efficient in space-constrained areas.
Network
Most enterprise edge use cases are run on-premises, either hardwired to a network or Wi-Fi. That makes the networking component essentially free. Remote devices that rely on cellular networks do incur cost based on the data streamed, which can get especially expensive if the data is video.
AI-on-5G is a key topic for consideration for many organizations, especially those that are looking at use cases that rely on guaranteed performance and high speed wireless. These solutions are still in the early stages of development, which makes cost difficult to determine.
Another networking consideration with edge computing is looking at what and how much data will be sent back to a data center or cloud. Most organizations use data from the edge to validate and retrain their AI models in a centralized location. Building a strategy around data that takes into consideration network and storage is critical to ensuring that the overall cost to maintain edge applications is managed.
Application costs
The number of AI applications in production is expected to grow to over 60% in the next few years. It’s not if an organization will deploy an AI application but when. Organizations either build or buy applications or use a hybrid approach.
Building AI applications
Building a data science team from scratch can be a large undertaking, especially due to the high demand and limited supply of qualified candidates in most locations. The average salary of a data scientist starts between $100-150K depending on skill level. Even organizations that have AI developers in-house often use a combination of build and buy strategy, saving their internal expertise for those critical applications that help them differentiate.
Buying AI applications
For organizations that do not already have data scientists and AI developers on staff, buying an AI application is the preferred method.
- Prebuilt applications can be customized and range anywhere from thousands to tens of thousands of dollars, depending on how they are licensed.
- Custom applications built from scratch can cost up to hundreds of thousands of dollars including development and roll-out.
Additional service contracts can be purchased for ongoing management and upgrades of these applications, depending on customer need.
Management costs
Edge computing presents unique challenges around management. These environments are highly distributed, deployed in remote locations without trained IT staff, and often lack the physical security expected from a data center.
Management software
Dedicated edge AI management solutions are most often priced based on usage, with systems or GPUs under management as the determining factor. These solutions have the benefit of key features that are tailored for edge deployments as well as the ability to scale as you grow in terms of cost. Some examples of these solutions include NVIDIA Fleet Command, Azure IoT, and AWS IoT.
Another management option is to extend traditional data center management solutions to the edge. Both VMware Tanzu and RedHat OpenShift are commonly found in many data center deployments, which means that IT teams have experience with them. Extending these solutions to the edge may require an increase in licensing cost, depending on the contract the company has.
Other costs that should be considered are the time it takes to make these solutions compatible with edge deployments, as well as the ongoing management of these environments.
Managed services
Some organizations look to outsource the management of their edge computing environments to system integrators or other management partners. These engagements can vary greatly, and include development of AI models, provisioning and management of infrastructure, as well as roll-out and updates of AI applications.
This option is typically considered when there is a limited amount of in-house expertise building and managing an edge AI solution. Depending on the scope, scale, and duration, these engagements cost anywhere from hundreds of thousands to millions of dollars.
Is edge computing cheaper than cloud computing?
Many organizations have made large investments in cloud computing. Now, with the rise of edge computing, they are looking for the cost savings. When comparing edge to cloud, edge AI is typically a new investment, so there is an upfront cost to getting started. When this cost is compared to the cost of moving streaming data and storing it in the cloud, there may be some reduced cost.
Most often, the move to edge computing is due to a use case that requires real-time responses or deployment in remote locations that have limited bandwidth. As an example, workloads like predictive maintenance, safety alerts, or autonomous machines would not be possible from a cloud environment due to the latency requirements.
When this is the case, it is not so important that the cost of edge computing is reduced; rather that the AI algorithm brings huge business value to the organization.
What is the value of edge computing?
Edge computing is a paradigm shift for most organizations. Like other transformational shifts, the process can be complex and expensive if not carefully thought through. However, when combined with AI, your organization can see huge benefits. From improved efficiency to reduced operating costs to improved customer intelligence and experience, the economic benefits that edge AI brings can be measured in millions of dollars.
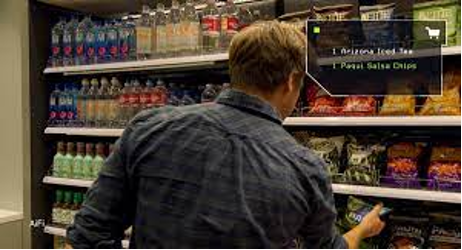
AI enables a frictionless shopping experience where customers can walk into a store, select the items they want to purchase, and leave with the items automatically charged to their account.
Retailers solve labor shortages and supply chain issues with AI: Over the last year retailers have experienced incredible challenges with the labor force being reduced by 6.2%, and shutdowns wreaking havoc on global supply chains. Using AI solutions, stores and restaurants have been able to improve automation, forecasting, and logistics to provide even better experiences to their customers.
AI inspection reduces total manufacturing cost: In any manufacturing line, manual inspection takes a significant amount of time and requires highly skilled workers to keep quality high. When accurate and quick defect detection is required, AI can be a perfect answer to increase overall equipment effectiveness (OEE) and boost production on a line. One manufacturer was able to reduce inspection costs from 30% of total manufacturing cost by using AI optical inspection in the factory.
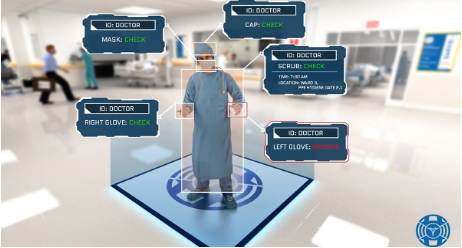
Smart hospitals optimize workflows and improve clinician experiences: The delivery of healthcare services is becoming more challenging, with providers, staff, and IT all having to do more with less resources. AI helps augment the work of these providers, giving them valuable and timely insights that not only reduce their burden but also save lives. Using vision AI to monitor patients and automate workflows, a 100-bed facility can save up to $11m annually.
Getting started
Given the value of edge AI, the topic of how to roll out a successful edge strategy is certainly be a key focus for organizations and IT departments. As a leader in AI, NVIDIA has worked with customers and partners to create edge computing solutions that deliver powerful, distributed compute; secure remote management; and compatibility with industry-leading technologies.
To understand if edge computing is the right solution for you, download the Considerations for Deploying AI at the Edge whitepaper.