This post features winners from the NVIDIA sponsored contest with Make: where makers submit their best robotics projects with a galactic theme.
Earlier this year, NVIDIA sponsored a contest with Make: Magazine, asking makers to submit their best AI-enabled droid projects with a galactic theme. Below are the two droid contest winners.
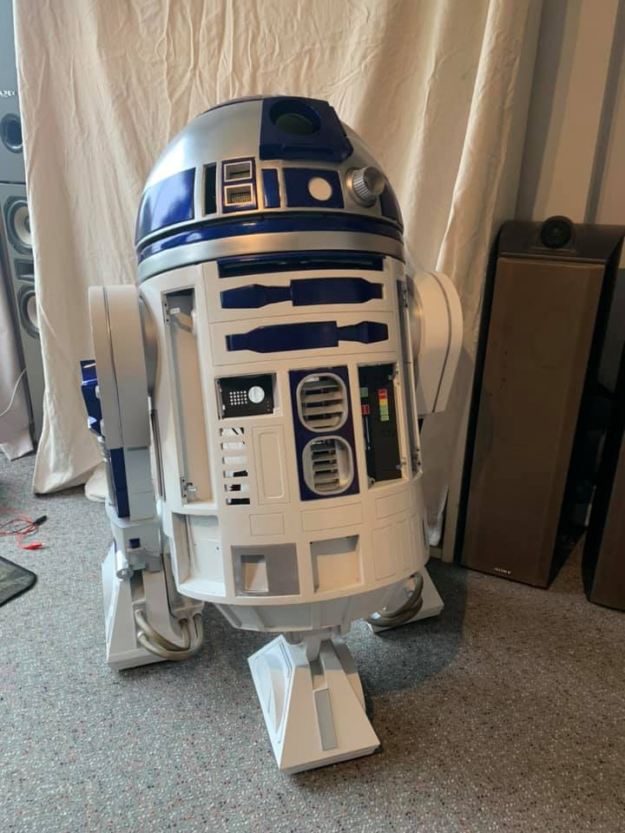
Autonomous 3D Printed R2-D2
During the Covid lockdown, John Ferguson looked for a fun project to build with his 11-year-old son. Using a 3D printer, John took on the massive task of creating every inch of his robot. About 40 kg of filament and 10 months of printing later, he had himself the body of an R2 unit. This hands-on project required sanding, prepping each piece, filling, painting, and tuning for a truly movie-grade finish.
A few components of the R2-D2 robot include Sabertooth Motor controllers, Sony cameras, two scooter motors, Arduino, NVIDIA Jetson Nano, a Muse EEG brainwave reader for an active periscope mechanism, and it is controlled with an Xbox 360 wireless controller. This project is a way for the Ferguson family to learn about AI, integrating NVIDIA Jetson Nano to activate R2’s vision-enabled object recognition capabilities and speech recognition.
“This is the first time we’ve done a project using object recognition and it’s a thrill. It really feels like the future is here! To teach children this capability and show them the interactivity—you really get stunned silence as a reaction,” said Ferguson.
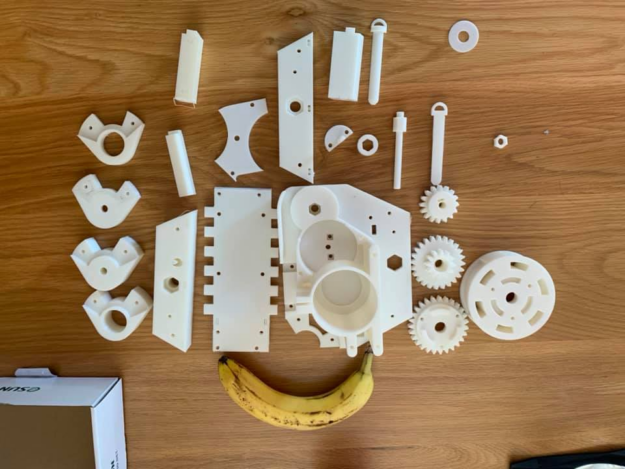
When asked why John decided to implement the NVIDIA Jetson Nano into his project, he said that the plastic body of the R2 is heavy, so having a lightweight component is preferable. It’s also a premium option for object recognition using AI, easily integrated with Python apps, and a good system for a young person to learn with.
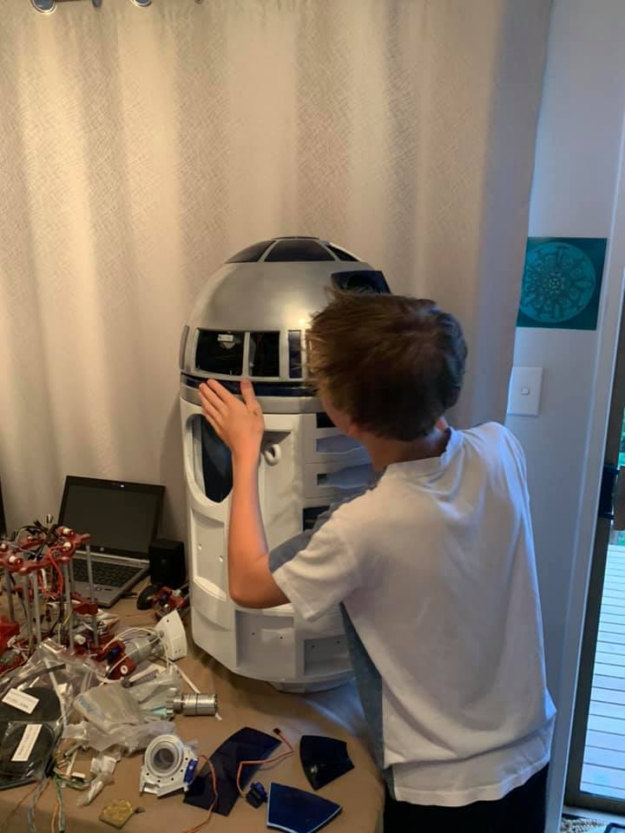
Their goal is to attend in-person events and have R2 autonomously strolling at their side using ROS2, identify other Star Wars characters accurately, and vocally respond just like the real thing. John and his son are building the body from scratch, training the recognition model using their own annotated image library, and optimizing the models.
“I’m not a developer. We’ve learned everything from videos and tutorials. We had the time and the passion, and that’s got us to where we are through experimenting and persistence,” Ferguson said.
At the heart of the R2-D2 project, John hopes to showcase the robot to local schools, outline his journey so that it’s replicable, and talk about the personal growth that comes with building something from scratch and having fun with robotics.
“I want to encourage young people to enjoy developing technology,” said Ferguson.
Follow along with “build log” updates on their Facebook page. >>
AI Made Accessible with RoboJango

Next up, we have Jim Nason’s impressive Mandalorian-inspired droid named RoboJango.
This droid is packed with features that any Star Wars fanatic would be psyched to see. To name a few, this droid has HD vision for eyes, acoustic sensors, dual lidar to promote autonomy, heat sensors, off-roading ATV capabilities, and a powerful winch for getting out of sticky situations. Similar to the R2-D2 robot, the RoboJango incorporates 3D printed parts mounted onto a wood frame and steel core.
RoboJango is surprisingly personable. It uses human-like movements and conversational AI skills, giving people in the room casual greetings, flexing its “muscles”, and spitting jokes. The RoboJango recognizes Jim’s family members, their pets, and harnesses object recognition capabilities by self-organizing to a DNN. This is done with several Arduinos, a battery matrix, customized software framework, and NVIDIA Jetson Nano as its brain.
The coolest thing about RoboJango is the maker, Jim Nason. With a 30-year professional history in programming, he started building this robot because his son asked for a 3D printer and wanted to build an android with the materials.

Three years ago, Jim started by just building a finger, then an arm, eventually leading to an AI-enabled robot. RoboJango also has functional anthropomorphic eyes, with a wire-based circulatory system based on a virtual representation of a human.
Talking with Jim, you get the sense that he really understands the mantra of being a maker, which is to dream, learn, and innovate:
“I’m teaching him how to play the ukulele and drums. Just need to work on the movements,” Nason said.

He also built a best friend for RoboJango, its very own robot dog.
Now, Jim wants to give back to the community and teach kids all about STEAM with his wacky and whimsical robotics projects. Since March of this year, Jim has taught over 1,500 virtual students across the United States and was awarded a 2021 Impact Award for his outstanding contributions to the classroom. On summer weekends, Jim hosted community builds in Long Beach where anyone could walk in and learn about robotics.
“RoboJango was created to drive funding for Long Island robotics apprentices. We want to teach to all communities and allow kids to have a ball,” said Nason
Learn more about Jim’s robotics course here, and follow his adventures on Instagram.
Thank you to our friends at Make: for hosting this contest.
To see more NVIDIA Jetson Nano projects, visit our Jetson community project page for inspiration.