Read about how deep learning models are used for an automated pick and place system, a feature more and more advanced warehouses are implementing.
Advanced warehouses process up to hundreds-of-thousands of orders a day. Fulfilling these quantities requires a lot of inventory, physical space, and complex workflows to support the high volume of items picked.
In addition, micro-fulfillment centers are becoming increasingly popular to fill same-day delivery orders from customers.
Operating these types of advanced facilities and machinery—along with a high volume of resources efficiently— requires skilled workers. While this workforce is shrinking, investing in edge computing and AI can help.
Shrinking Workforce
Modern warehouses feature robust automation. Autonomous machines and vehicles move products around warehouses, conveyor systems move goods into shipping containers, and use advanced 3D grids to provide pick-and-sort systems that pack products intelligently. But even with the current level of automation, modern warehouses are still labor intensive.
If boxes are different sizes, automated packing is difficult and often done manually. Some items are large and heavy, requiring a forklift and a driver to move. Finding and retaining employees is a challenge and COVID-19 protocols have exacerbated the problem. And while the workforce is shrinking, the warehouse industry is growing rapidly. The next generation of warehouses needs to focus on automation to succeed.
Deep Learning for Advanced Industry
Advancements in deep learning and edge computing provide intelligence and automate more processes of warehouses. Automated pick and place systems are an example of an operation advanced warehouses are implementing. In a pick and place system, an autonomous machine identifies an object among other objects in a bin, then selects, and places that object for packing elsewhere.
For this system to be automated, many different deep learning models are required. That’s because some objects are very hard to detect with computer vision, like translucent, reflective, or non-uniform objects.
The walkthrough below outlines how deep learning models are used for an automated pick and place system.
First, the object grasp point needs to be identified. This is the simplest deep learning model, but an important function for automation. Once it is known where to grasp an object, it is necessary to understand how the object exists in 3D space. Therefore, a depth estimation model is needed that allows the pick and place system to understand the depth of the object in the environment.
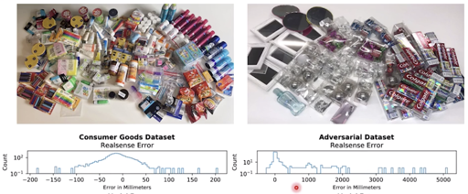
Once the object has been picked, it needs to be placed. To do this, an orientation model is used to determine the position in space of the item picked.
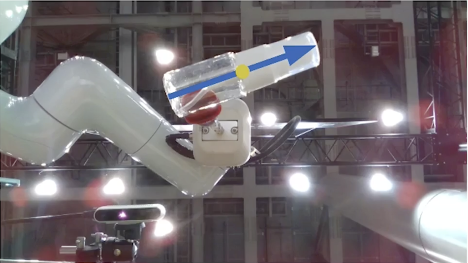
Combining those models and others allows for effective bin packing.

Pick and place systems showcase just some examples of models being used by retailers to improve warehouse automation.
To learn more about deep learning for pick and place systems as well as other models involved in modern warehouses, check out Deep Learning in Robotic Automation and Warehouse Logistics.