This is the last installment of the series of articles on the RAPIDS ecosystem with this being the ninth installment. The series explores and discusses various aspects of RAPIDS that allow its users solve ETL (Extract, Transform, Load) problems, build ML (Machine Learning) and DL (Deep Learning) models, explore expansive graphs, process signal and system … Continued
This is the last installment of the series of articles on the RAPIDS ecosystem with this being the ninth installment. The series explores and discusses various aspects of RAPIDS that allow its users solve ETL (Extract, Transform, Load) problems, build ML (Machine Learning) and DL (Deep Learning) models, explore expansive graphs, process signal and system log, or use SQL language via BlazingSQL to process data.
Today’s interconnected world makes us more vulnerable to cyber attacks: ever-present IoT devices record and listen to what we do, spam and phishing emails threaten us every day, and attacks on networks that steal data can lead to serious consequences. These systems produce terabytes of logs full of information that can help detect and protect vulnerable systems. Estimating on the conservative side, a medium-sized company with hundreds to thousands of interconnected devices can produce upwards of 100GB of log files per day. Also, the rate of events that get logged can reach levels counted in tens of thousands per second.
CLX (pronounced clicks) is part of the RAPIDS ecosystem that accelerates the processing and analysis of cyber logs. As part of RAPIDS, it builds on top of RAPIDS DataFrames cuDF, and further extends the capabilities of the RAPIDS ML library cuML by tapping into the latest advances in natural language processing field to organize unstructured data and build classification models.
The previous posts in the series showcased other areas:
- In the first post, python pandas tutorial we introduced cuDF, the RAPIDS DataFrame framework for processing large amounts of data on an NVIDIA GPU.
- The second post compared similarities between cuDF DataFrame and pandas DataFrame.
- In the third post, querying data using SQL we introduced BlazingSQL, a SQL engine that runs on GPU.
- In the fourth post, data processing with Dask we introduced a Python distributed framework that helps to run distributed workloads on GPUs.
- In the fifth post, the functionality of cuML, we introduced the Machine Learning library of RAPIDS.
- In the sixth post, the use of RAPIDS cuGraph, we introduced a GPU framework for processing and analyzing cyber logs.
- In the seventh post, the functionality of cuStreamz, we introduced how GPUs can be used to process streaming data.
- In the eighth post, the functionality of RAPIDS cuSignal, we introduced how to accelerate signal processing in python.
In this post, we introduce CLX. To help with getting familiar with CLX, we also published a cheat sheet that can be downloaded here CLX-cheatsheet, and an interactive notebook with all the current functionality of CLX showcased here.
Cybersecurity
With the advent of personalized computers, the adversarial games shifted from pure reconnaissance missions and traditional warfare to interrupting the computer systems of one’s enemy. The organizations like the National Security Agency (NSA) are full of scientists of various backgrounds that daily try to keep our national networks safe so an adversary cannot access the power grid or our banking system. At this level stakes are exceedingly high as are the defense mechanisms to prevent such attacks.
Personal computers or business networks are a different measure: while an attack on a single computer or a network might not cripple or otherwise threaten the well-being of citizens, it can have profound effects on one person’s or a business’s finances and/or future opportunities.
Many of these attacks leave a trace, a breadcrumbs trail of information that can help a business detect an attack so it can defend itself against it. After all, any attack that compromises the ability of a company to conduct a business as usual leads to lost productivity. Worse yet, if an attacker gains access to and steals intellectual property, it may cripple or completely ruin such a business.
However, how can a business that generates upwards of 100GB per day of logs keep up with all this data flood?
cyBERT
Historically, the approach was to parse the logs using Regex. And while we’re big fans of Regex per se, such an approach becomes impractical if a business needs to maintain thousands of different patterns for every single type of a log such a business collects. And that’s where it all begins: in order to detect attacks on our network we need data, and in order to get data we need to parse logs. Without data we cannot train any machine learning model. No other way around it.
BERT (or Bi-directional Encoder Representations from Transformers) model is a deep neural network introduced by Google to build a better understanding of natural language. Unlike previous approaches for solving problems in the NLP field that relied on recurrent network architectures (like LSTM – Long Short-Term Memory), the BERT model is a feed-forward network that learns the context of a word by scanning a sentence in both directions. Thus, BERT would produce a different embedding (or numerical representation) for similarly sounding sentences, like ‘She is watching TV’ and ‘She is watching her kids grow’.
In the context of cybersecurity logs, such embedding can be helpful to distinguish between IP addresses, network endpoints, ports, or free flow comments or messages. In fact, by using BERT embeddings one can train a model to detect such entities. Enter cyBERT!
cyBERT is an automatic tool to parse logs and extract relevant information. To get started, we just need to load the model we intend to use:
cybert = Cybert() cybert.load_model( 'pytorch_model.bin' , 'config.json' )
The pytorch_model.bin
is a PyTorch model that was trained to recognize entities from Apache WebServer logs; it can be downloaded from the models.huggingface.co/bert/raykallen/cybert_apache_parser
S3 bucket. In the same bucket we can find the config.json
file.
Once we have the model loaded, CyBERT will utilize the power of NVIDIA GPUs to parse the logs at rapid speed to extract the useful information and produce a structured representation of the log information. The API makes it really simple.
logs_df = cudf.read_csv(‘apache_log.csv') parsed_df, confidence_df = cybert.inference(logs_df["raw"])
The first dataframe returned contains all the parsed fields from the logs.
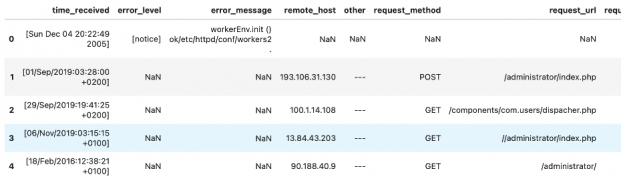
while the confidence_df
DataFrame outlines how confident the CyBERT model is about each extracted piece of information.
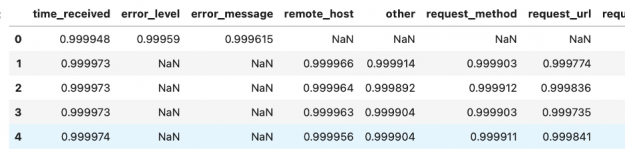
As you can see the model is pretty confident and a glimpse at the data confirms that the extracted information matches the column name.
Want to try other functionality of CLX or simply run through the above examples? Go to the CLX cheatsheet here!