Posted by Han Zhang, Research Scientist and Jing Yu Koh, Software Engineer, Google Research
Automatic text-to-image synthesis, in which a model is trained to generate images from text descriptions alone, is a challenging task that has recently received significant attention. Its study provides rich insights into how machine learning (ML) models capture visual attributes and relate them to text. Compared to other kinds of inputs to guide image creation, such as sketches, object masks or mouse traces (which we have highlighted in prior work), descriptive sentences are a more intuitive and flexible way to express visual concepts. Hence, a strong automatic text-to-image generation system can also be a useful tool for rapid content creation and could be applied to many other creative applications, similar to other efforts to integrate machine learning into the creation of art (e.g., Magenta).
State-of-the-art image synthesis results are typically achieved using generative adversarial networks (GANs), which train two models — a generator, which tries to create realistic images, and a discriminator, which tries to determine if an image is real or fabricated. Many text-to-image generation models are GANs that are conditioned using text inputs in order to generate semantically relevant images. This is significantly challenging, especially when long, ambiguous descriptions are provided. Moreover, GAN training can be prone to mode collapse, a common failure case for the training process in which the generator learns to produce only a limited set of outputs, so that the discriminator fails to learn robust strategies to recognize fabricated images. To mitigate mode collapse, some approaches use multi-stage refinement networks that iteratively refine an image. However, such systems require multi-stage training, which is less efficient than simpler single-stage end-to-end models. Other efforts rely on hierarchical approaches that first model object layouts before finally synthesizing a realistic image. This requires the use of labeled segmentation data, which can be difficult to obtain.
In “Cross-Modal Contrastive Learning for Text-to-Image Generation,” to appear at CVPR 2021, we present the Cross-Modal Contrastive Generative Adversarial Network (XMC-GAN), which addresses text-to-image generation by learning to maximize the mutual information between image and text using inter-modal (image-text) and intra-modal (image-image) contrastive losses. This approach helps the discriminator to learn more robust and discriminative features, so XMC-GAN is less prone to mode collapse even with one-stage training. Importantly, XMC-GAN achieves state-of-the-art performance with a simple one-stage generation, as compared to previous multi-stage or hierarchical approaches. It is end-to-end trainable, and only requires image-text pairs (as opposed to labeled segmentation or bounding box data).
Contrastive Losses for Text-to-Image Synthesis
The goal of text-to-image synthesis systems is to produce clear, photo-realistic scenes with high semantic fidelity to their conditioned text descriptions. To achieve this, we propose to maximize the mutual information between the corresponding pairs: (1) images (real or generated) with a sentence describing the scene; (2) a generated image and a real image with the same description; and (3) regions of an image (real or generated) and words or phrases associated with them.
In XMC-GAN, this is enforced using contrastive losses. Similar to other GANs, XMC-GAN contains a generator for synthesizing images, and a discriminator that is trained to act as a critic between real and generated images. Three sets of data contribute to the contrastive loss in this system — the real images, the text that describes those images, and the images generated from the text descriptions. The individual loss functions for both the generator and the discriminator are combinations of the loss calculated from whole images with the full text description, combined with the loss calculated from sub-divided images with associated words or phrases. Then, for each batch of training data, we calculate the cosine similarity score between each text description and the real images, and likewise, between each text description and the batch of generated images. The goal is for the matching pairs (both text-to-image and real image-to-generated image) to have high similarity scores and for non-matching pairs to have low scores. Enforcing such a contrastive loss allows the discriminator to learn more robust and discriminative features.
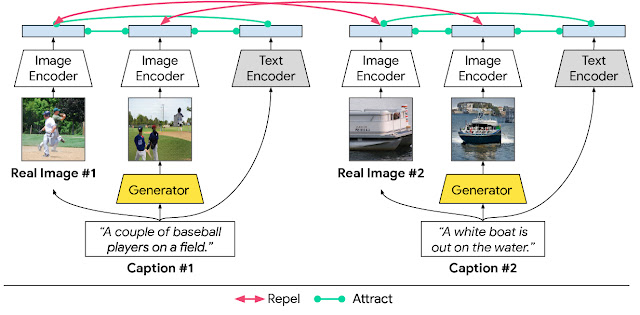 |
Inter-modal and intra-modal contrastive learning in our proposed XMC-GAN text-to-image synthesis model. |
Results
We apply XMC-GAN to three challenging datasets — the first was a collection of MS-COCO descriptions of MS-COCO images, and the other two were datasets annotated with Localized Narratives, one of which covers MS-COCO images (which we call LN-COCO) and the other of which describes Open Images data (LN-OpenImages). We find that XMC-GAN achieves a new state of the art on each. The images generated by XMC-GAN depict scenes that are of higher quality than those generated using other techniques. On MS-COCO, XMC-GAN improves the state-of-the-art Fréchet inception distance (FID) score from 24.7 to 9.3, and is significantly preferred by human evaluators.
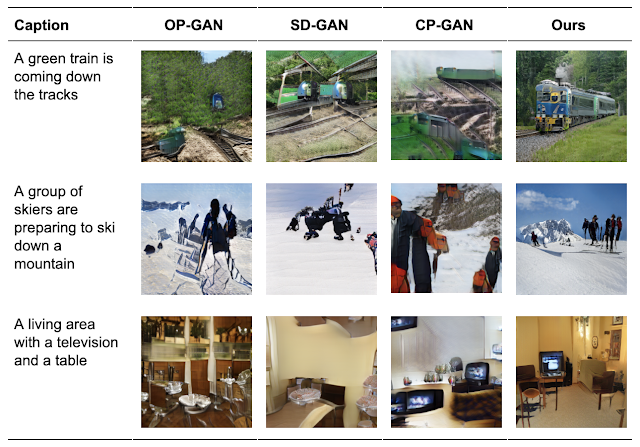 |
Selected qualitative results for generated images on MS-COCO. |
Similarly, human raters prefer the image quality in XMC-GAN generated images 77.3% of the time, and 74.1% prefer its image-text alignment compared to three other state-of-the-art approaches (CP-GAN, SD-GAN, and OP-GAN) .
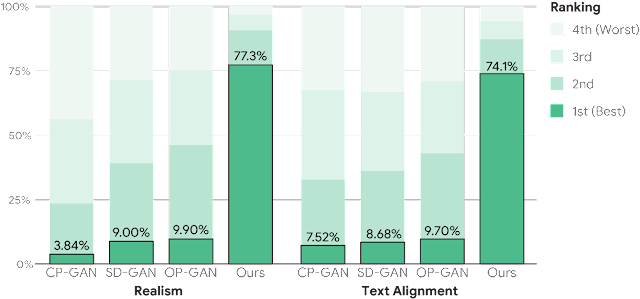 |
Human evaluation on MS-COCO for image quality and text alignment. Annotators rank (anonymized and order-randomized) generated images from best to worst. |
XMC-GAN also generalizes well to the challenging Localized Narratives dataset, which contains longer and more detailed descriptions. Our prior work TReCS tackles text-to-image generation for Localized Narratives using mouse trace inputs to improve image generation quality. Despite not receiving mouse trace annotations, XMC-GAN is able to significantly outperform TReCS on image generation on LN-COCO, improving state-of-the-art FID from 48.7 to 14.1. Incorporating mouse traces and other additional inputs into an end-to-end model such as XMC-GAN would be interesting to study in future work.
In addition, we also train and evaluate on the LN-OpenImages, which is more challenging than MS-COCO because the dataset is much larger with images that cover a broader range of subject matter and that are more complex (8.4 objects on average). To the best of our knowledge, XMC-GAN is the first text-to-image synthesis model that is trained and evaluated on Open Images. XMC-GAN is able to generate high quality results, and sets a strong benchmark FID score of 26.9 on this very challenging task.
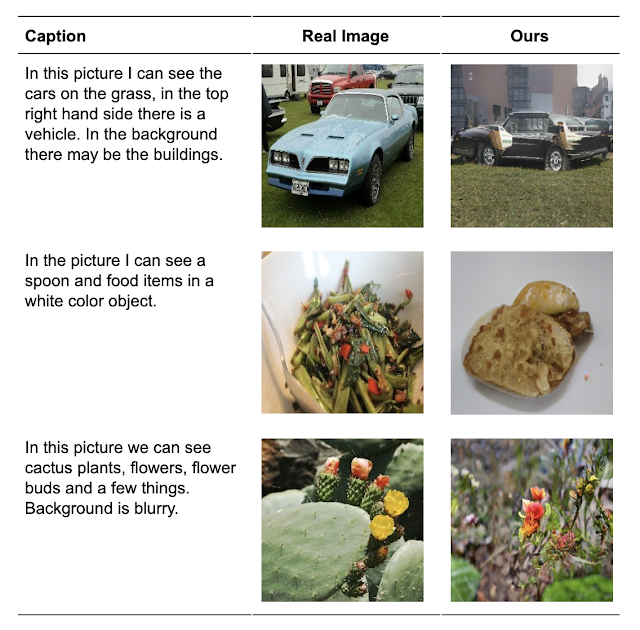 |
Random samples of real and generated images on Open Images. |
Conclusion and Future Work
In this work, we present a cross-modal contrastive learning framework to train GAN models for text-to-image synthesis. We investigate several cross-modal contrastive losses that enforce correspondence between image and text. For both human evaluations and quantitative metrics, XMC-GAN establishes a marked improvement over previous models on multiple datasets. It generates high quality images that match their input descriptions well, including for long, detailed narratives, and does so while being a simpler, end-to-end model. We believe that this represents a significant advance towards creative applications for image generation from natural language descriptions. As we continue this research, we are continually evaluating responsible approaches, potential applications and risk mitigation, in accordance with our AI Principles.
Acknowledgements
This is a joint work with Jason Baldridge, Honglak Lee, and Yinfei Yang. We would like to thank Kevin Murphy, Zizhao Zhang, Dilip Krishnan for their helpful feedback. We also want to thank the Google Data Compute team for their work on conducting human evaluations. We are also grateful for general support from the Google Research team.
